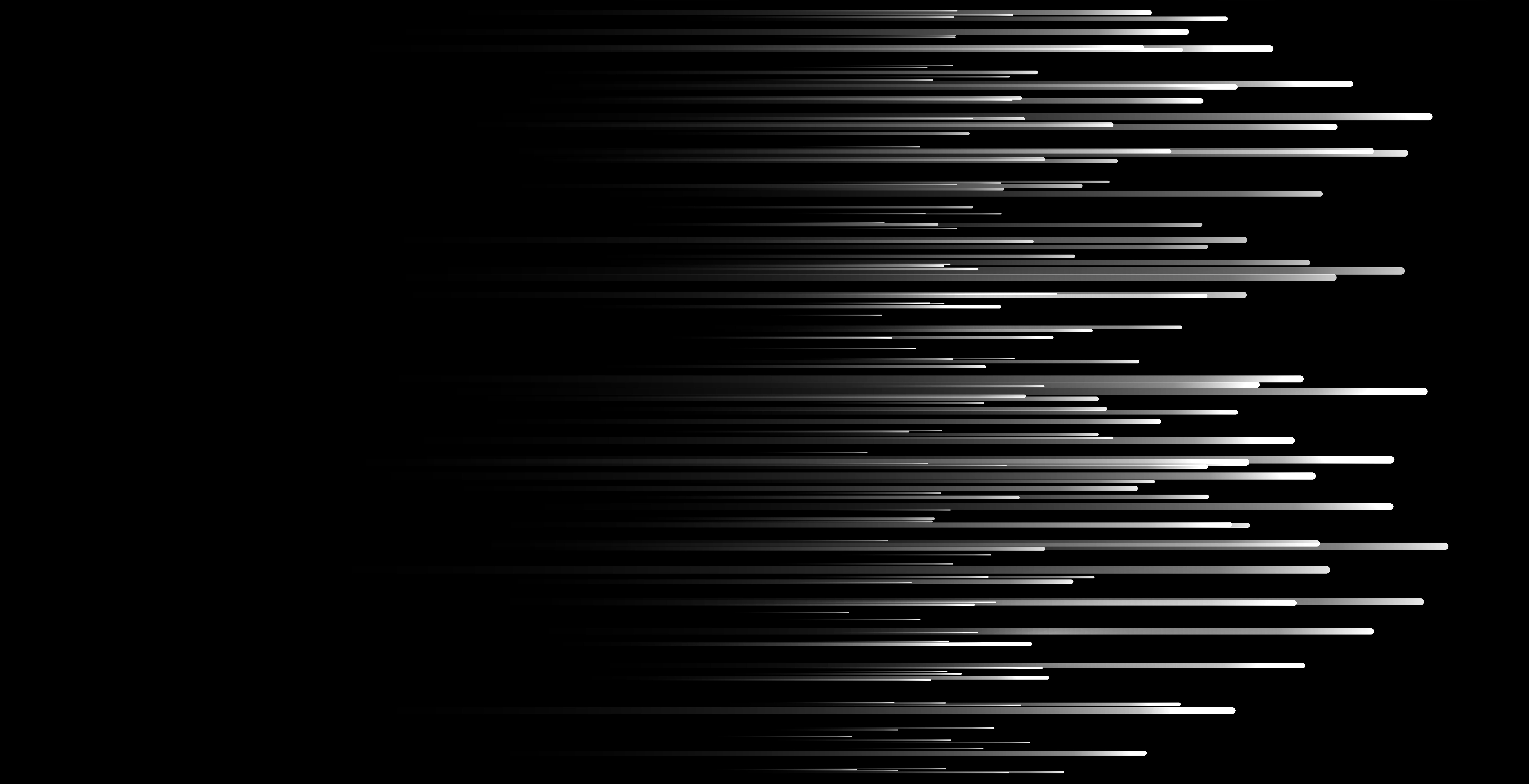
the awareness of data analytic tools
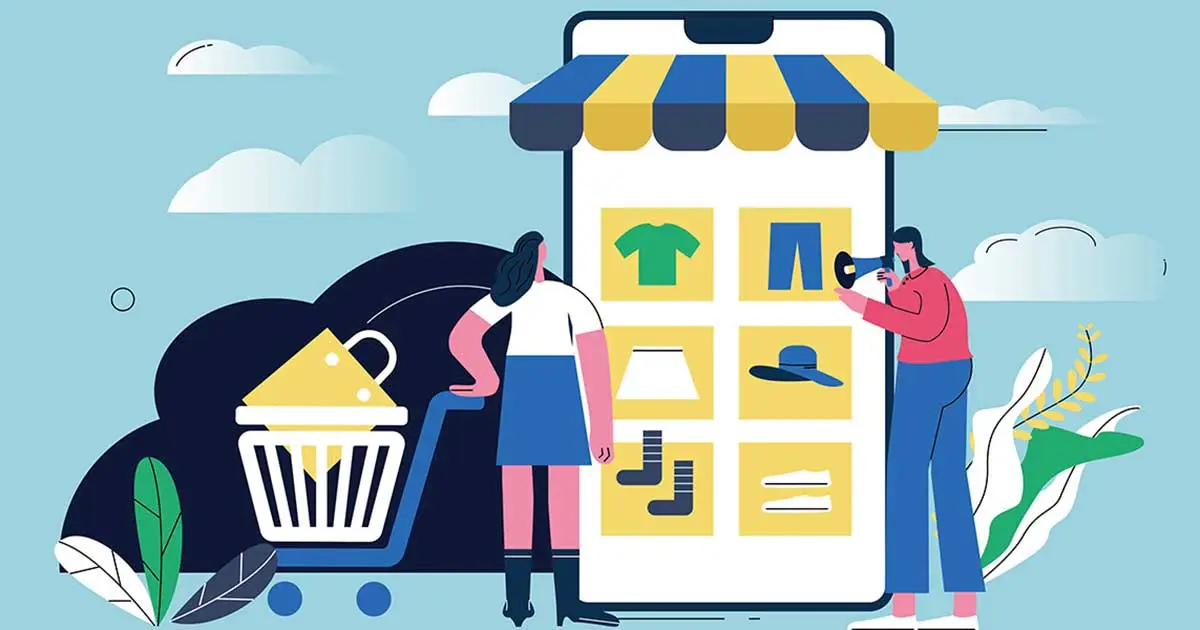
THE AWARENESS OF DATA ANALYTIC TOOLS
What is data analytics Data analytics is the process of analyzing raw data in order to make conclusions about that information. Alternatively, it is the process of inspecting, cleansing, transforming and modeling data with the aim of discovering useful information, suggesting conclusions and supporting decision making. These qualitative and quantitative techniques and processes enhance productivity and business gain and also allow us to make informed decisions disregarding guessing. The tool or software used in realizing these processes is called the analytic tool. Why Data Analytics Matters Data analytics is important because it helps businesses optimize their performances. Implementing it into the business model means companies can help reduce costs by identifying more efficient ways of doing business and by storing large amounts of data. A company can also use data analytics to make better business decisions and help analyze customer trends and satisfaction which can lead to new and better products and services. Data Analytics is no more a nice option in the running of business but has become an integral part of businesses. In this era of technological advancement and the rapid growth of AI, it has become imperative for businesses to analyze transactional data to enable them make critical decisions, identify income or revenue leakages that may go unnoticed. Types of Data analytics The main goal of data analytics is to help organizations make smarter decisions for better business outcomes. Data analytics is broken down into four basics types Descriptive, Diagnostic, Predictive and Prescriptive Analytics Descriptive analytics As the name implies, it summarizes raw data and convert it into a form that can be easily understood. Descriptive analytics answers the question of what happened over a given period of time. E.g. Has the number of views gone up? Are sales stronger this year than last year? This is perhaps the most used type of analytics. Diagnostic analytics This focuses more on why something happened. It allows the analyst to dig deeper into an issue so that they can arrive at the source of a problem. E.g. Did the weather affect beer sales? Did the latest marketing campaign impact sales? Predictive analytics Predictive analytics helps businesses to forecast trends based on the current events and suggests a course of action. It uses the findings of descriptive and diagnostic analytics to detect clusters and exceptions, and to predict future trends, which makes it a valuable tool for forecasting. E.g. If the likelihood of a hot summer is measured as an average of these five weather models is above 58%, we should add an evening shift to the brewery and rent an additional tank to increase output Prescriptive Analytics This type of analytics explains the step-by-step process in a situation. It literally prescribe what action to take to eliminate a future problem or take full advantage of a promising trend. For instance, a prescriptive analysis is what comes into play when a company is able to identify opportunities for repeat purchases based on customer analytics and sales history. Steps to Data analytics The process involved in data analysis involves several different steps: (Where and how did we come to the steps below)
1. Undertstand the Business / Define the Business Need This stage involves defining the need of the organization by interacting with the people in the organization whose processes or business you aim to improve with data. Clearly define the problem you aim to solve with the analysis, identify the sources of data, set metrics to track along the way.
2. Data Collection With the business need clearly defined, it's time to collect the data that will be used in the analysis. The first step is to determine the data requirements or how the data is grouped. Data may be separated by age, demographic, income or gender. Data values may be numerical or be divided by category. Data can be taken various sources depending on the organization or the needs of the project. Some sources of data are: Primary source: Data can be collected from the organizations internal sources o. Usually, this is gathered from the CRM software, ERP system, Core Banking Application, marketing automation tools, and others. The IT team of the organization will make it available or setup a private database for you. Secondary source: While it may not be required to gather from secondary sources, it is sometimes useful. Some sources include; review sites, social media APIs, economic trends APIs, google analytics, google trends, depending on the business requirements of the analysis.
3. Data Cleaning / Preparation Once you've gotten your data from all the necessary sources, you will have to clean and sort through it. Data preparation is crucial to the quality and accuracy of results generated. This step usually involves, purging duplicate data, removing unwanted data, masking sensitive data, restricting, and removing any other inconsistencies that could skew the analysis. This step helps correct any errors before it goes on to a data analyst to be analyzed.
4. Analyze Data After you've collect the data and cleaned it time to perform analysis on it. A software like Arbutus Analyzer can be used to perform analysis on the data collected. Data can be analyzed as graphs, pivot table, summary table, etc.
5. Interpret the Results The final step is interpreting the results from the data analysis. After going through all the stages of data analytics, you can make better decisions for the business or agency because your choices are backed by data that has been robustly collected and analyzed. Importance of Data analytics: Helps companies to spend their advertising budget with maximum effect. Objective answers that can put an end to an argument Identify the important trends Identify business performance problems that require some sort of action and provide corrective measures. Providing data analysis in a visual manner, which leads to faster and better decisions Better awareness regarding the habits of potential customers Providing companies with an edge over their competitors Data analytics is no longer a nice option, it's the core of the business.
AI systems can learn and adapt from their experiences. This allows them to perform tasks that may be impossible or impractical for traditional computer programs. The rise of AI can be attributed to advancements in hardware, such as GPUs and TPUs, as well as advancements in machine learning algorithms and techniques.
One of the most promising areas of AI is Natural Language Processing (NLP). NLP is a subfield of machine learning that focuses on enabling computers to understand, interpret, and generate human language. Examples of NLP applications include virtual assistants like Apple's Siri and Amazon's Alexa, and language translation services like Google Translate.
As AI continues to advance, its potential impact on society is immense. It has the potential to revolutionize various industries, including healthcare, finance, and transportation. AI could also help address some of the world's most pressing challenges, such as climate change, poverty, and inequality.
Related Blog Posts
- Nov 01, 2021
Why Revenue Assurance
It involves a process or software solution that enables an organisation to monitor all..
Read More - Dec 13, 2023
Preparing Data For Analysis
Analyzer is the flagship analytics product offered by Arbutus that support desktop based
Read More - June 10, 2023
A Guide to Igniting Your Imagination
Lorem ipsum dolor sit amet Artificial Intelligence (AI) is a branch of computer science that seeks to create intelligent machines.
Read More